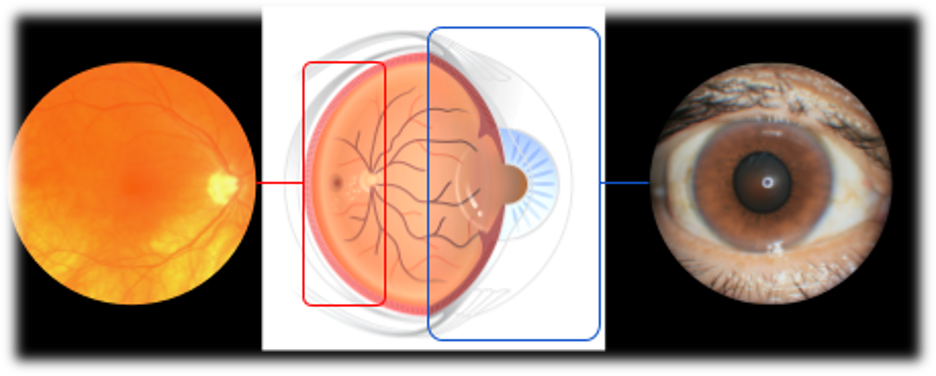
AI Healthcare : Detecting Signs of Disease from External Images of the Eye
Introduction
A recent study demonstrated that diabetic retinopathy, diabetic macular edoema, poor blood glucose management, and high lipid levels may all be detected using deep-learning models trained on external pictures of the eye. The diagnosis process and patient outcomes might both be significantly improved by this technology. How It Works Retinal fundus photographs can provide valuable information about a patient's systemic health, but they require specialized equipment and trained professionals to capture and analyze the images. In contrast, external photographs of the eye can be taken with common equipment and potentially from home, making this technology more accessible and cost-effective.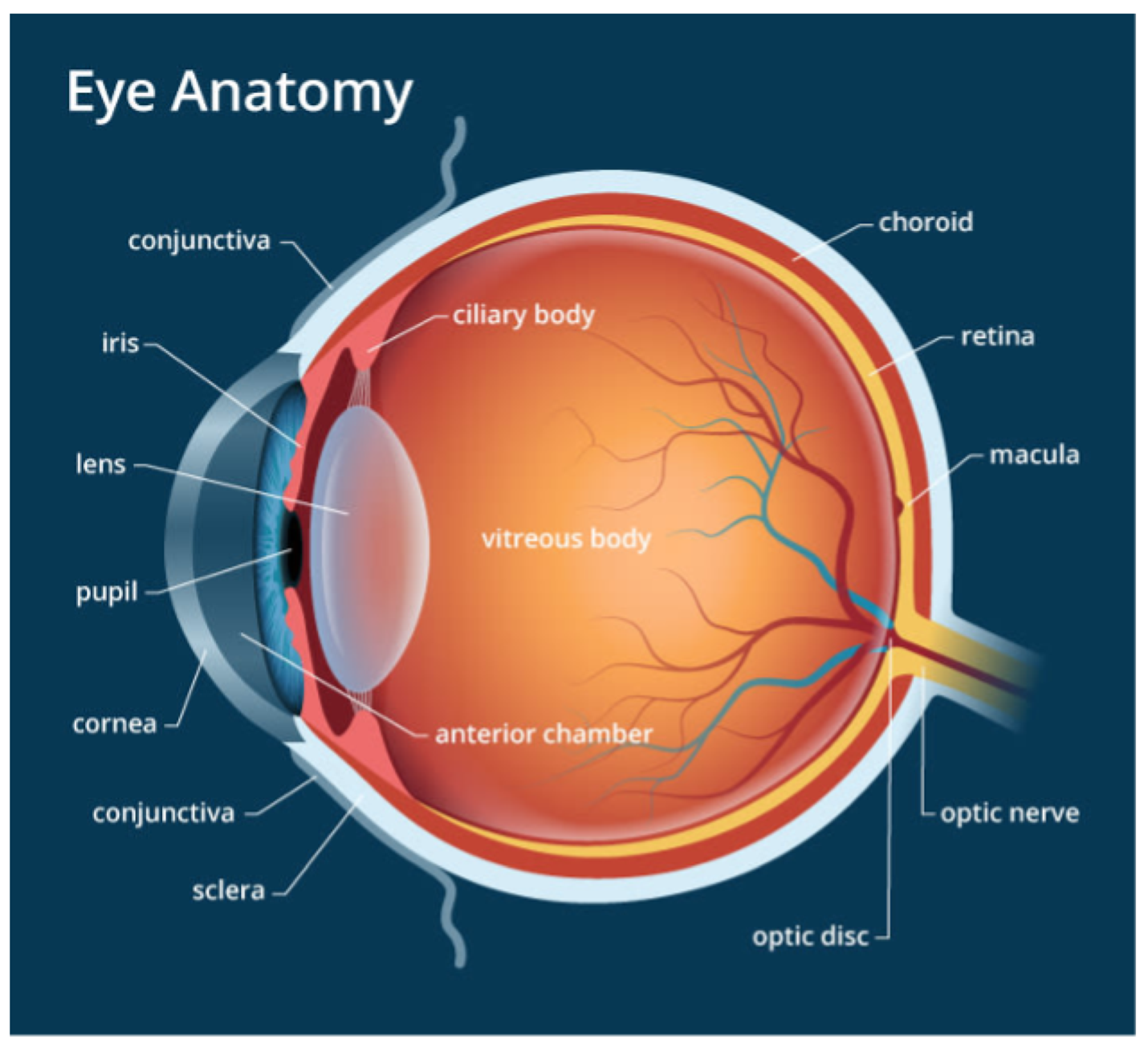
Comparison to Traditional Methods
The study discovered that, when utilizing self-reported demographic and medical history data, the predictive performance of deep-learning models was noticeably greater than the performance of logistic regression models. This technology may enable early intervention and better patient outcomes by delivering speedier and more precise findings.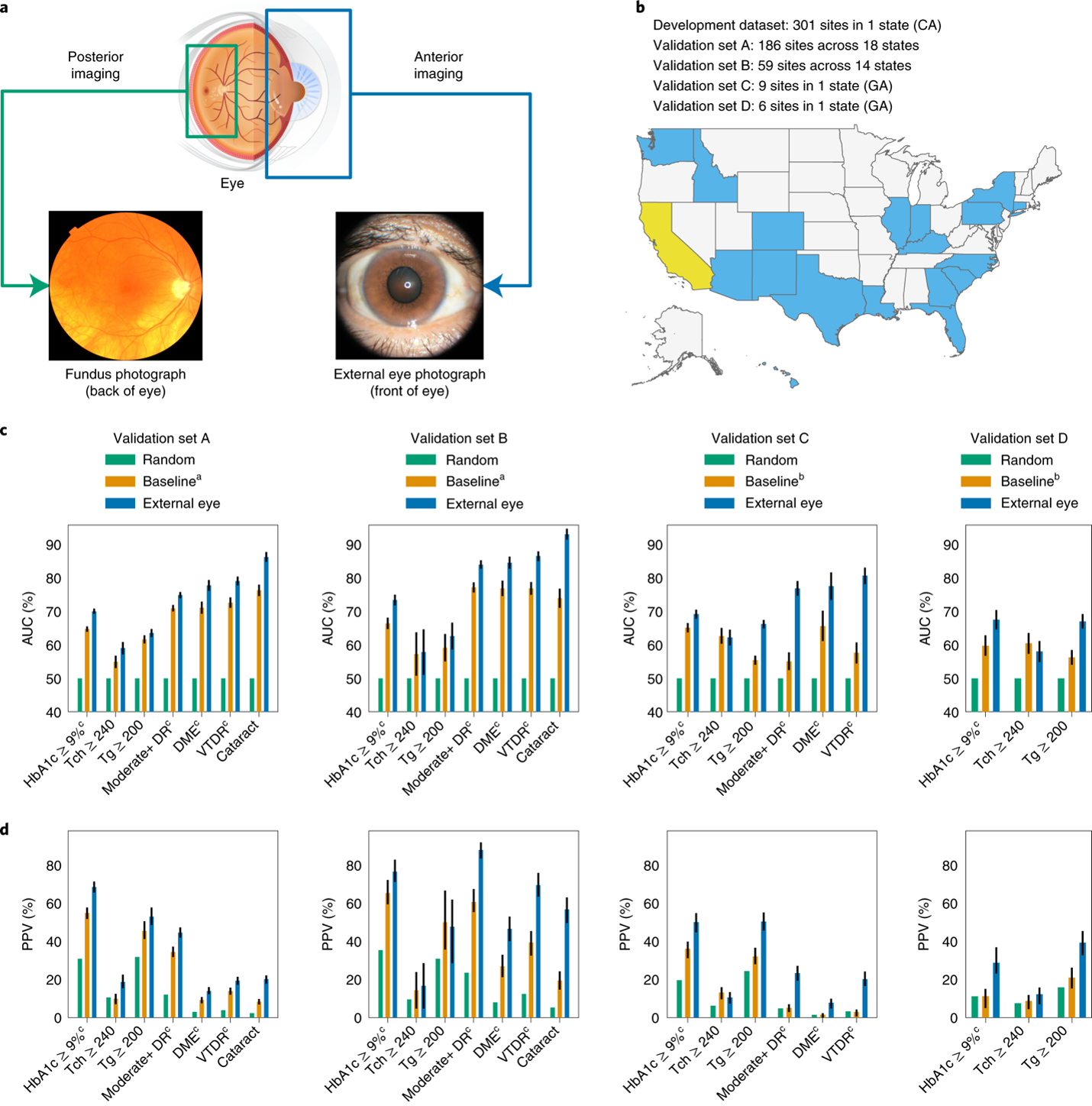
The process typically involves training AI models on large datasets of medical images with annotations provided by healthcare professionals. These models can then analyze new images and flag potential areas of concern, assisting healthcare providers in making more accurate diagnoses. AI can speed up the diagnostic process, reduce human error, and extend healthcare services to underserved areas where access to specialists may be limited. A little example about image classification how we can adapt it to health care or precision diagnostic: https://poloclub.github.io/cnn-explainer. It's important to note that while AI can be a valuable tool in disease detection, it should always be used in conjunction with the expertise of trained healthcare professionals who can interpret the AI-generated results and make informed treatment decisions. Additionally, regulatory approvals and clinical validation are critical before AI-based diagnostic tools can be widely deployed in healthcare settings to ensure their safety and effectiveness.
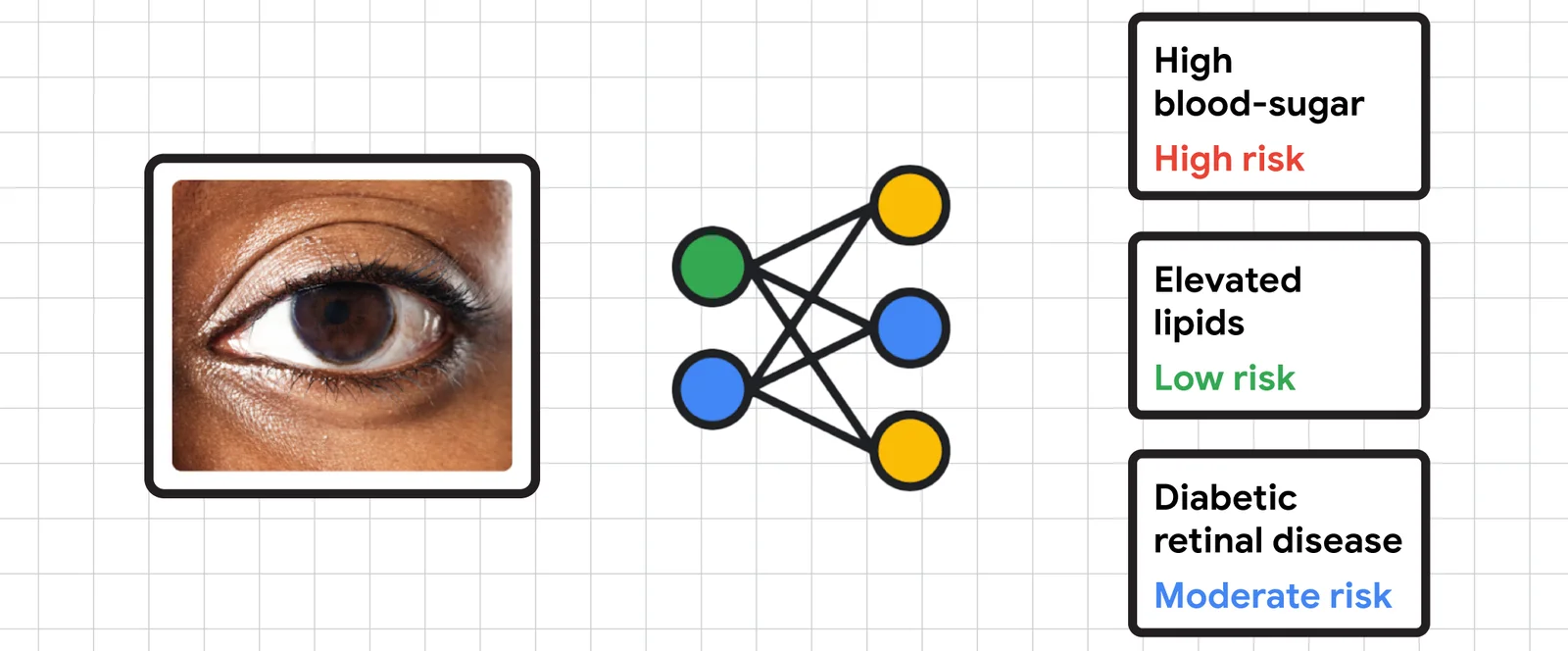